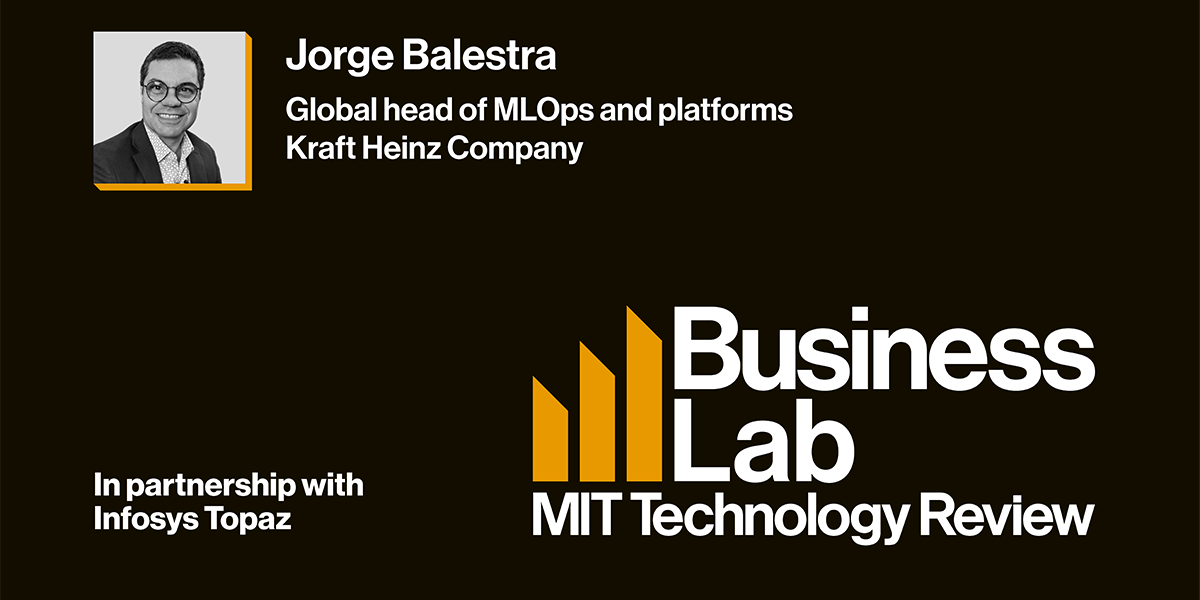
Jorge: Certainly. My role, I will call, has two major focuses in two areas. One of them is I lead the machine learning engineering operations of the company globally. And on the other hand, I provide all of the analytical platforms that the company is using also on a global basis. So in role number one in my machine learning engineering and operations, what my team does is we grab all of these models that our community of data scientists that are working globally are coming up with, and we grabbed them and we strengthened it. Our major mission here is the first thing we need to do is we need to make sure that we are applying engineering practices to make them production ready and they can scale, they can also run in a cost-effective manner, and from there we ensure that in my operations hat they are there when needed.
So a lot of these models, because they become part of our day-to-day operations, they’re going to come with certain specific service level commitments that we need to make, so my team makes sure that we are delivering on those with the right expectations. And on my other hand, which is the analytical platforms, is that we do a lot of descriptive, predictive, and prescriptive work in terms of analytics. The descriptive portion where you’re talking about just the regular dashboarding, summarization piece around our data and where the data lives, all of those analytical platforms that the company is using are also something that I take care of. And with that, you would think that I have a very broad base of customers in the company both in terms of geographies where they are from some of our businesses in Asia, all the way to North America, but also across the organization from marketing to HR and everything in between.
Going into your other question about how machine learning is helping our consumers in the grocery aisle, I’ll probably summarize that for a CPG it’s all about having the right product at the right price, at the right location for you. What that means is on the right product, their machine learning can help a lot of our marketing teams, for example, even when they are now with the latest generative AI capabilities are showing up like brainstorming and creating new content to R&D, what we’re trying to figure out what is the best formulas for our products, there’s definitely now ML is making inroads in that space, the right price, all about cost efficiencies throughout from our plans to our distribution centers, making sure that we are eliminating waste. Leveraging machine learning capabilities is something that we are doing across the board from our revenue management, which is the right price for people to buy our products.
And then last but not least is the right location. So we need to make sure that when our consumers are going into their stores or are buying our products online that the product is there for you and you’re going to find the product you like, the flavor you like immediately. And so there is a huge effort around predicting our demand, organizing our supply chain, our distribution, scheduling our plans to make sure that we are producing the right quantities and delivering them to the right places so our consumers can find our products.
Laurel: Well, that certainly makes sense since data does play such a crucial role in deploying advanced technologies, especially machine learning. So how does Kraft Heinz ensure the accessibility, quality and security of all of that data at the right place at the right time to drive effective machine learning operations or MLOps? Are there specific best practices that you’ve discovered?
Jorge: Well, the best practice that I can probably advise people on is definitely data is the fuel of machine learning. So without data, there is no modeling. And data, organizing your data, both the data that you have internally and externally takes time. Making sure that it’s not only accessible and you are organizing it in a way that you don’t have a gazillion technologies to deal with is important, but also I would say the curation of it. That is a long-term commitment. So I strongly advise anyone that is listening right now to understand that your data journey, as it is, is a journey, it doesn’t have an end destination, and also it’s going to take time.
And the more you are successful in terms of getting all the data that you need organized and making sure that is available, the more successful you’re going to be leveraging all of that with models in machine learning and great things that are there to actually then accomplish a specific business outcome. So a good metaphor that I like to say is there’s a lot of researchers, and MIT is known for its research, but the researchers cannot do anything without the librarians, with all the people that’s organizing the knowledge around so you can go and actually do what you need to do, which is in this case research. Never forget that data is the fuel, and data, it takes effort, it is a journey, it never ends, because that’s what is really what I would call what differentiates a lot of successful efforts compared to unsuccessful ones.
Laurel: Getting back to that right place at the right time mentality, within the last few years, the consumer packaged goods, or you mentioned earlier, the CPG sector, has seen such major shifts from changing customer demands to the proliferation of e-commerce channels. So how can AI and machine learning tools help influence business outcomes or improve operational efficiency?
Recent Comments