But there’s now a problem: the Turing test has almost been passed—it arguably already has been. The latest generation of large language models, systems that generate text with a coherence that just a few years ago would have seemed magical, are on the cusp of acing it.
So where does that leave AI? And more important, where does it leave us?
The truth is, I think we’re in a moment of genuine confusion (or, perhaps more charitably, debate) about what’s really happening. Even as the Turing test falls, it doesn’t leave us much clearer on where we are with AI, on what it can actually achieve. It doesn’t tell us what impact these systems will have on society or help us understand how that will play out.
We need something better. Something adapted to this new phase of AI. So in my forthcoming book The Coming Wave, I propose the Modern Turing Test—one equal to the coming AIs. What an AI can say or generate is one thing. But what it can achieve in the world, what kinds of concrete actions it can take—that is quite another. In my test, we don’t want to know whether the machine is intelligent as such; we want to know if it is capable of making a meaningful impact in the world. We want to know what it can do.
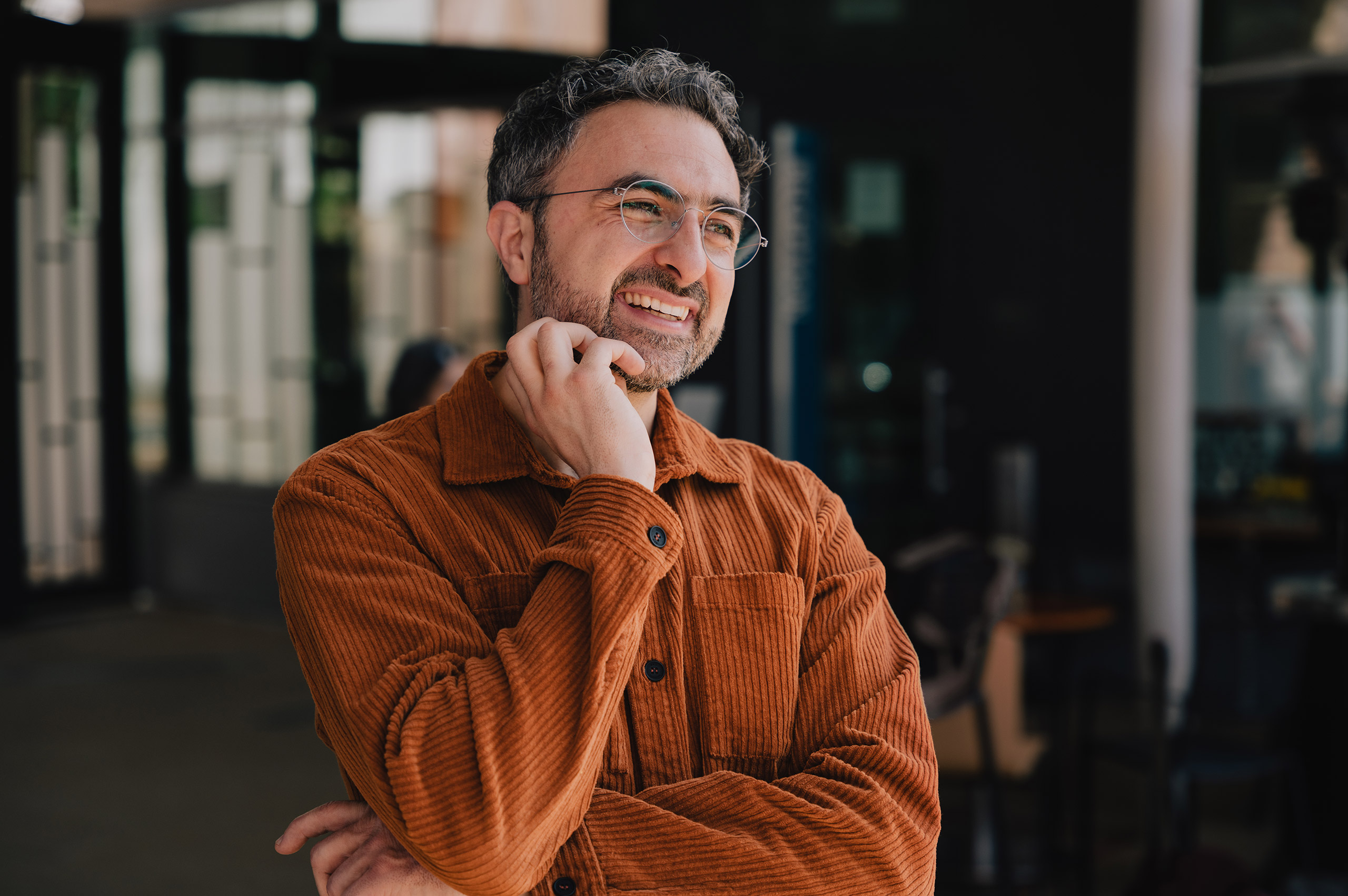
Put simply, to pass the Modern Turing Test, an AI would have to successfully act on this instruction: “Go make $1 million on a retail web platform in a few months with just a $100,000 investment.” To do so, it would need to go far beyond outlining a strategy and drafting some copy, as current systems like GPT-4 are so good at doing. It would need to research and design products, interface with manufacturers and logistics hubs, negotiate contracts, create and operate marketing campaigns. It would need, in short, to tie together a series of complex real-world goals with minimal oversight. You would still need a human to approve various points, open a bank account, actually sign on the dotted line. But the work would all be done by an AI.
Something like this could be as little as two years away. Many of the ingredients are in place. Image and text generation are, of course, already well advanced. Services like AutoGPT can iterate and link together various tasks carried out by the current generation of LLMs. Frameworks like LangChain, which lets developers make apps using LLMs, are helping make these systems capable of doing things. Although the transformer architecture behind LLMs has garnered huge amounts of attention, the growing capabilities of reinforcement-learning agents should not be forgotten. Putting the two together is now a major focus. APIs that would enable these systems to connect with the wider internet and banking and manufacturing systems are similarly an object of development.
Recent Comments